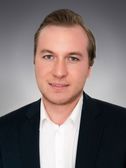
Sebastian Erhardt, M.Sc.
Doktorand und wissenschaftlicher Mitarbeiter
Innovation and Entrepreneurship Research
+49 89 24246-589
sebastian.erhardt(at)ip.mpg.de
Arbeitsbereiche
Datenwissenschaften, Künstliche Intelligenz, Maschinelles Lernen, Natürliche Spracherkennung und -verarbeitung, Innovationsökonomie
Wissenschaftlicher Werdegang
Seit 2020
Doktorand und Wissenschaftlicher Mitarbeiter am Max-Planck-Institut für Innovation und Wettbewerb (Innovation and Entrepreneurship Research)
Seit 2020
Postgraduales Studium Betriebswirtschaftliche Forschung (MBR), Ludwig-Maximilians-Universität (LMU) München
02/2020 - 07/2020
Gast Blockchain-Wissesnschaftler, Liphardt Lab ‒ Stanford Distributed Trust Initiative, Leland Stanford Junior University
10/2019 - 05/2021
Master of Science (M.Sc.) in Informatik, Ludwig-Maximilians-Universität (LMU) München
08/2018 - 07/2020
Honours Degree in Technology Management, Center for Digital Technology and Management (CDTM), Ludwig-Maximilians-Universität (LMU) München und Technische Universität München (TUM)
10/2017 - 04/2019
Master of Science (M.Sc.) in Wirtschaftsinformatik, Technische Universität München (TUM)
10/2014 - 03/2017
Bachelor of Science (B.Sc.) in Wirtschaftsinformatik, Technische Universität München (TUM)
Beruflicher Werdegang
2/2005 - heute
Selbständiger Entwickler/Berater ‒ Freiberufliche Tätigkeit für kleine, mittlere und große Unternehmen sowie für den öffentlichen Sektor, Deutschland
08/2020 - 10/2020
Entwickler/Produktmanager ‒ Tech4Germany, Bundesministerium des Innern, für Bau und Heimat, Bundesministerium der Finanzen und Informationstechnikzentrum Bund, Berlin
02/2016 - 03/2017
Werkstudent, Deloitte Digital, München
09/2015 - 02/2016
Werkstudent, Deloitte Consulting, München
Ehrungen, Stipendien
Seit 2019
Fellow des Tech4Germany Programms der Bundesregierung, eine Initiative unter der Schirmherrschaft von Bundesminister Prof. Dr. Helge Braun, Chef des Bundeskanzleramts
2018 - 2019
Think Digital Stipendium des Internet Business Cluster (IBC) e.V.
Seit 2018
Mitglied des Elitenetzwerks Bayern, eine Initiative des Freistaats Bayern zur Förderung des wissenschaftlichen Nachwuchses
2016 - 2018
Manage&More Stipendium der UnternehmerTUM
Publikationen
Logic Mill - A Knowledge Navigation System, CEUR Workshop Proceedings 3775, 25-35.
(2024).- Logic Mill is a scalable and openly accessible software system that identifies semantically similar documents within either one domain-specific corpus or multi-domain corpora. It uses advanced Natural Language Processing (NLP) techniques to generate numerical representations of documents. It leverages a large pre-trained language model to generate these document representations. The system focuses on scientific publications and patent documents and contains more than 200 million
documents. It is easily accessible via a simple Application Programming Interface (API) or via a web interface. Moreover, it is continuously being updated and can be extended to text corpora from other domains. We see this system as a general-purpose tool for future research applications in the social sciences and other domains. - https://ceur-ws.org/Vol-3775/paper7.pdf
- Also published as: arXiv preprint 2301.00200
- Event: 5th Workshop on Patent Text Mining and Semantic Technologies (PatentSemTech 2024) co-located with the 47th International ACM SIGIR Conference on Research and Development in Information Retrieval (SIGIR 2024), Washington D.C., 2024-10-24
PaECTER: Patent-level Representation Learning using Citation-informed Transformers, arXiv preprint 2402.19411. DOI
(2024).- PaECTER is a publicly available, open-source document-level encoder specific for patents. We fine-tune BERT for Patents with examiner-added citation information to generate numerical representations for patent documents. PaECTER performs better in similarity tasks than current state-of-the-art models used in the patent domain. More specifically, our model outperforms the next-best patent specific pre-trained language model (BERT for Patents) on our patent citation prediction test dataset on two different rank evaluation metrics. PaECTER predicts at least one most similar patent at a rank of 1.32 on average when compared against 25 irrelevant patents. Numerical representations generated by PaECTER from patent text can be used for downstream tasks such as classification, tracing knowledge flows, or semantic similarity search. Semantic similarity search is especially relevant in the context of prior art search for both inventors and patent examiners. PaECTER is available on Hugging Face.
Logic Mill - A Knowledge Navigation System, arXiv preprint 2301.00200.
(2022).- Logic Mill is a scalable and openly accessible software system that identifies semantically similar documents within either one domain-specific corpus or multi-domain corpora. It uses advanced Natural Language Processing (NLP) techniques to generate numerical representations of documents. Currently it leverages a large pre-trained language model to generate these document representations. The system focuses on scientific publications and patent documents and contains more than 200 million documents. It is easily accessible via a simple Application Programming Interface (API) or via a web interface. Moreover, it is continuously being updated and can be extended to text corpora from other domains. We see this system as a general-purpose tool for future research applications in the social sciences and other domains.
- https://doi.org/10.48550/arXiv.2301.00200
- Also published in: CEUR Workshop Proceedings 3775
Vorträge
07.12.2023
Logic Mill - A Knowledge Navigation System
2nd CESifo / ifo Junior Workshop on Big Data
Ort: München
02.12.2023
Logic Mill - A Knowledge Navigation System
Innovation Information Initiative Technical Working Group Meeting - National Bureau of Economic Research
Ort: Cambridge, MA, US
09.06.2022
Logic Mill
Munich Summer Institute
Ort: München
08.04.2022
Tracing the Flow of Knowledge from Science to Technology Using Deep Learning
European Patent Office ARP Program
Ort: München
04.11.2020
Introduction to Git & Github
Max-Planck-Institut für Innovation und Wettbewerb
Ort: online