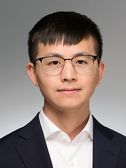
Cheng Li, M.Sc.
Doktorand und wissenschaftlicher Mitarbeiter
Innovation and Entrepreneurship Research
+49 89 24246-453
cheng.li(at)ip.mpg.de
Arbeitsbereiche:
Innovationsverbreitung, Innovationsstrategie, Immaterialgüterrechte, Komplexe Netzwerke, Künstliche Intelligenz
Wissenschaftlicher Werdegang
Seit 06/2023
Doktorand und Wissenschaftlicher Mitarbeiter am Max-Planck-Institut für Innovation und Wettbewerb
(Innovation and Entrepreneurship Research)
2018 – 2021
Master of Science, Robotics, Systems and Control
Titel der Masterarbeit: Data-Driven Analysis of the “Ranking Quality” Network Formation Model
ETH Zürich, Schweiz
2015 – 2018
Bachelor of Science, Maschinenbau
Technische Universität Dresden
Beruflicher Werdegang
01/2022 – 05/2023
Patentingenieur
Wuesthoff & Wuesthoff, München
07/2020 – 02/2021
Studentische Hilfskraft
ETH Zürich, Schweiz
09/2019 – 02/2020
Forschungspraktikum
Huawei, Zürich, Schweiz
09/2016 – 01/2018
Teaching Assistant
TU Dresden
Ehrungen, Stipendien, wissenschaftliche Preise
04/2018
DAAD STIBET Graduiertenstipendium
Publikationen
Artikel in referierten Fachzeitschriften
A Meritocratic Network Formation Model for the Rise of Social Media Influencers, Nature Communications 2021.
(2021).- Many of today’s most used online social networks such as Instagram, YouTube, Twitter, or Twitch are based on User-Generated Content (UGC). Thanks to the integrated search engines, users of these platforms can discover and follow their peers based on the UGC and its quality. Here, we propose an untouched meritocratic approach for directed network formation, inspired by empirical evidence on Twitter data: actors continuously search for the best UGC provider. We theoretically and numerically analyze the network equilibria properties under different meeting probabilities: while featuring common real-world networks properties, e.g., scaling law or small-world effect, our model predicts that the expected in-degree follows a Zipf’s law with respect to the quality ranking. Notably, the results are robust against the effect of recommendation systems mimicked through preferential attachment based meeting approaches. Our theoretical results are empirically validated against large data sets collected from Twitch, a fast-growing platform for online gamers.
- https://doi.org/10.1038/s41467-021-27089-8