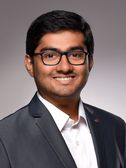
Mainak Ghosh, M.Sc.
Doktorand und wissenschaftlicher Mitarbeiter
Innovation and Entrepreneurship Research
+49 89 24246-561
mainak.ghosh(at)ip.mpg.de
Persönliche Website:
Arbeitsbereiche:
Natürliche Spracherkennung und -verarbeitung, Innovationsökonomik, Science of Science, Immaterialgüterrechte
Wissenschaftlicher Werdegang
Seit 03/2020
Wissenschaftlicher Mitarbeiter und Doktorand, Max-Planck-Institut für Innovation und Wettbewerb (Innovation and Entrepreneurship Research)
10/2017 - 11/2019
Master of Science (M.Sc.) im Studiengang “Data Engineering and Analytics”, Technische Universität München (TUM); Titel der Masterarbeit: “Multilingual Opinion Mining on Social Media Comments Using Unsupervised Neural Clustering Methods”
11/2017 - 02/2018
Studentischer Mitarbeiter, Max-Planck-Institut für Sozialrecht und Sozialpolitik, München
05/2013 - 07/2013
Forschungspraktikum, Indian Statistical Institute, Kalkutta, Indien
06/2012 - 07/2012
Praktikum, Globsyn Business School, Kalkutta, Indien
2010 - 2014
Bachelor of Engineering (B.E.) im Studiengang “Computer Science & Technology”, Indian Institute of Engineering Science & Technology, Shibpur, Indien
Beruflicher Werdegang
03/2018 - 03/2020
Werkstudent, IDS GmbH – Analysis and Reporting Services (IDS), München
08/2014 - 09/2017
Softwareingenieur, Acclaris Business Solutions Pvt Ltd, Kalkutta, Indien
Wissenschaftliche Preise und Ehrungen
2013
Zertifikat CCS (Cognizant Certified Student), IT Foundation Skills
2009
Auszeichnung, Mathematischer Kompetenztest, Association for Improvement of Mathematics Teaching (AIMT), Kalkutta, Indien
2008
Zertifikat für besondere Leistungen in Physikwissenschaften und Mechanik
Publikationen
Artikel in referierten Fachzeitschriften
Human Mediation Leads to Higher Compliance in Digital Mental Health: Field Evidence from India, Frontiers in Behavioral Economics, 2. DOI
(2023).Konferenzbeiträge
Logic Mill - A Knowledge Navigation System, CEUR Workshop Proceedings 3775, 25-35.
(2024).- Logic Mill is a scalable and openly accessible software system that identifies semantically similar documents within either one domain-specific corpus or multi-domain corpora. It uses advanced Natural Language Processing (NLP) techniques to generate numerical representations of documents. It leverages a large pre-trained language model to generate these document representations. The system focuses on scientific publications and patent documents and contains more than 200 million
documents. It is easily accessible via a simple Application Programming Interface (API) or via a web interface. Moreover, it is continuously being updated and can be extended to text corpora from other domains. We see this system as a general-purpose tool for future research applications in the social sciences and other domains. - https://ceur-ws.org/Vol-3775/paper7.pdf
- Also published as: arXiv preprint 2301.00200
- Event: 5th Workshop on Patent Text Mining and Semantic Technologies (PatentSemTech 2024) co-located with the 47th International ACM SIGIR Conference on Research and Development in Information Retrieval (SIGIR 2024), Washington D.C., 2024-10-24
SocialVisTUM: An Interactive Visualization Toolkit for Correlated Neural Topic Models on Social Media Opinion Mining, in: Galia Angelova et al. (
- Recent research in opinion mining proposedword embedding-based topic modeling methods that provide superior coherence compared to traditional topic modeling. In this paper, we demonstrate how these methods can be used to display correlated topic models on social media texts using SocialVisTUM, our proposed interactive visualization toolkit. It displays a graph with topics as nodes and their correlations as edges. Further details are displayed interactively to support the exploration of large text collections, e.g., representative words and sentences of topics, topic and sentiment distributions, hierarchical topic clustering, and customizable, predefined topic labels. The toolkit optimizes automatically on custom data for optimal coherence. We show a working instance of the toolkit on data crawled from English social media discussions about organic food consumption. The visualization confirms findings of a qualitative consumer research study. SocialVisTUM and its training procedures are accessible online.
- Event: International Conference "Recent Advances in Natural Language Processing, Shoumen, 2021-09-01
Andere Veröffentlichungen, Presseartikel, Interviews
Proceedings of the 12th Conference on Language Resources and Evaluation (LREC 2020), Marseille, 1376-1381.
(2020). An Evaluation of Progressive Neural Networks for Transfer Learning in Natural Language Processing, in:- A major challenge in modern neural networks is the utilization of previous knowledge for new tasks in an effective manner, otherwise known as transfer learning. Fine-tuning, the most widely used method for achieving this, suffers from catastrophic forgetting. The problem is often exacerbated in natural language processing (NLP). In this work, we assess progressive neural networks (PNNs) as an alternative to fine-tuning. The evaluation is based on common NLP tasks such as sequence labeling and text classification. By gauging PNNs across a range of architectures, datasets, and tasks, we observe improvements over the baselines throughout all experiments.
- Conference Paper
- Conference Volume
- Event: 12th Language Resources and Evaluation Conference, Marseille, 2020-05-11
Diskussionspapiere
PaECTER: Patent-level Representation Learning using Citation-informed Transformers, arXiv preprint 2402.19411. DOI
(2024).- PaECTER is a publicly available, open-source document-level encoder specific for patents. We fine-tune BERT for Patents with examiner-added citation information to generate numerical representations for patent documents. PaECTER performs better in similarity tasks than current state-of-the-art models used in the patent domain. More specifically, our model outperforms the next-best patent specific pre-trained language model (BERT for Patents) on our patent citation prediction test dataset on two different rank evaluation metrics. PaECTER predicts at least one most similar patent at a rank of 1.32 on average when compared against 25 irrelevant patents. Numerical representations generated by PaECTER from patent text can be used for downstream tasks such as classification, tracing knowledge flows, or semantic similarity search. Semantic similarity search is especially relevant in the context of prior art search for both inventors and patent examiners. PaECTER is available on Hugging Face.
Logic Mill - A Knowledge Navigation System, arXiv preprint 2301.00200.
(2022).- Logic Mill is a scalable and openly accessible software system that identifies semantically similar documents within either one domain-specific corpus or multi-domain corpora. It uses advanced Natural Language Processing (NLP) techniques to generate numerical representations of documents. Currently it leverages a large pre-trained language model to generate these document representations. The system focuses on scientific publications and patent documents and contains more than 200 million documents. It is easily accessible via a simple Application Programming Interface (API) or via a web interface. Moreover, it is continuously being updated and can be extended to text corpora from other domains. We see this system as a general-purpose tool for future research applications in the social sciences and other domains.
- https://doi.org/10.48550/arXiv.2301.00200
- Also published in: CEUR Workshop Proceedings 3775
Vorträge
19.09.2023
Patent Quality - Measurement & Analysis
Forschungsseminar
Ort: Schloss Ringberg
27.02.2023
Patent Quality - Measurement & Analysis
Forschungsseminar
Ort: Frauenchiemsee
23.09.2023
Logic Mill
Summer School on Data and Algorithms for Science, Technology & Innovation Studies, KU Leuven
Ort: Leuven, Belgien
07.09.2022
Logic Mill
Forschungsseminar
Ort: Bernried am Starnberger See
11. – 14.04.2022
Logic Mill & Hierarchical Embedding
Forschungssemiar
Ort: Ohlstadt
01.12.2021
Logic Mill: Patent Embedding
Forschungsseminar
Ort: Schloss Ringberg
01.10.2021
Logic Mill: Automation of Patent Full-Text Collection
Forschungsseminar
Ort: Feldkirchen-Westerham
23.03.2021
Logic Mill - Citation Prediction
Forschungsseminar
Ort: online
23.03.2021
Automation and Mental Health Platform Design: Field Experiment Plan
Forschungsseminar
Ort: online
10.09.2020
Knowledge Mining, Digitalization, Machine Learning
Forschungsseminar
Ort: online (München)